Impact of Economic Indicators on Real Estate Prices
Software Engineering
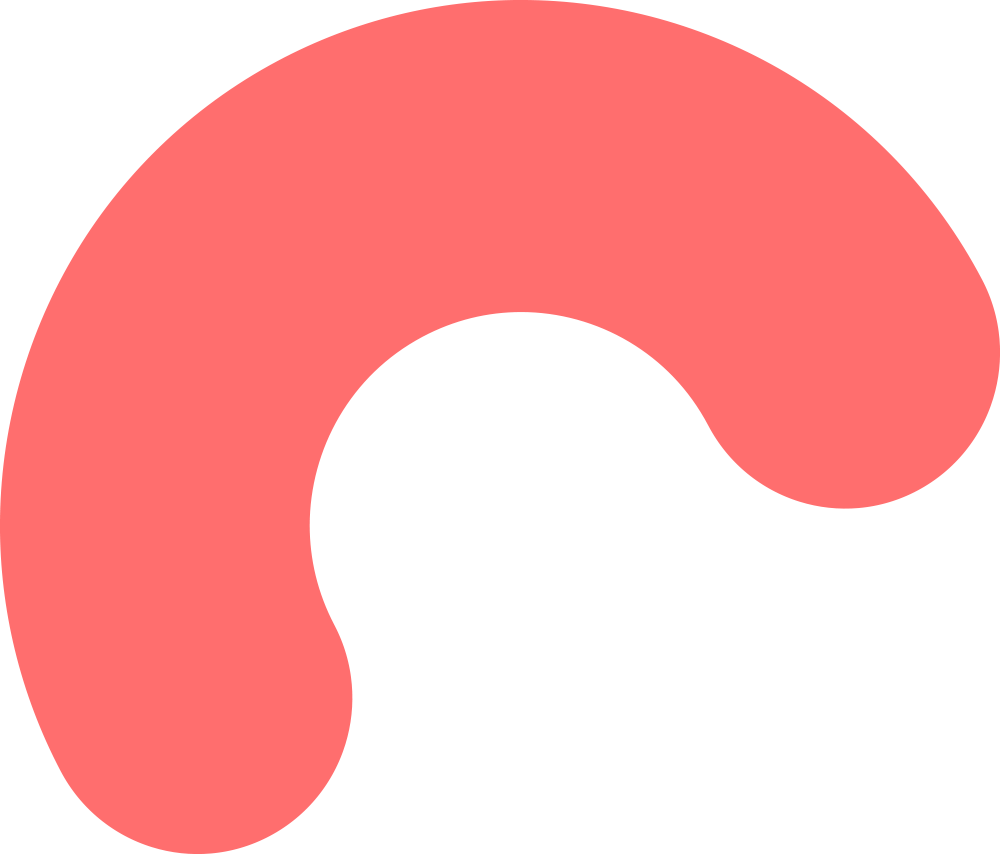
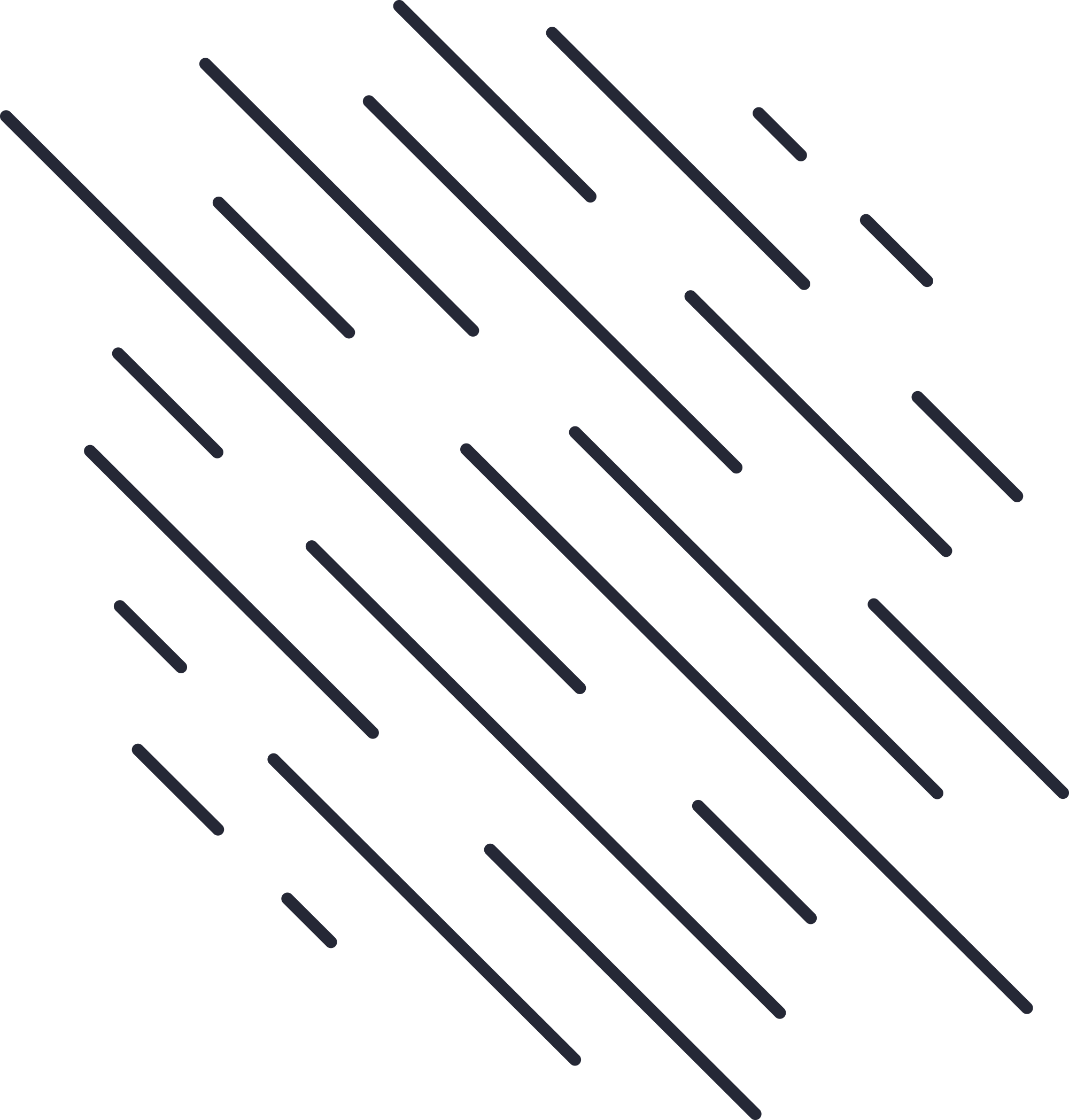
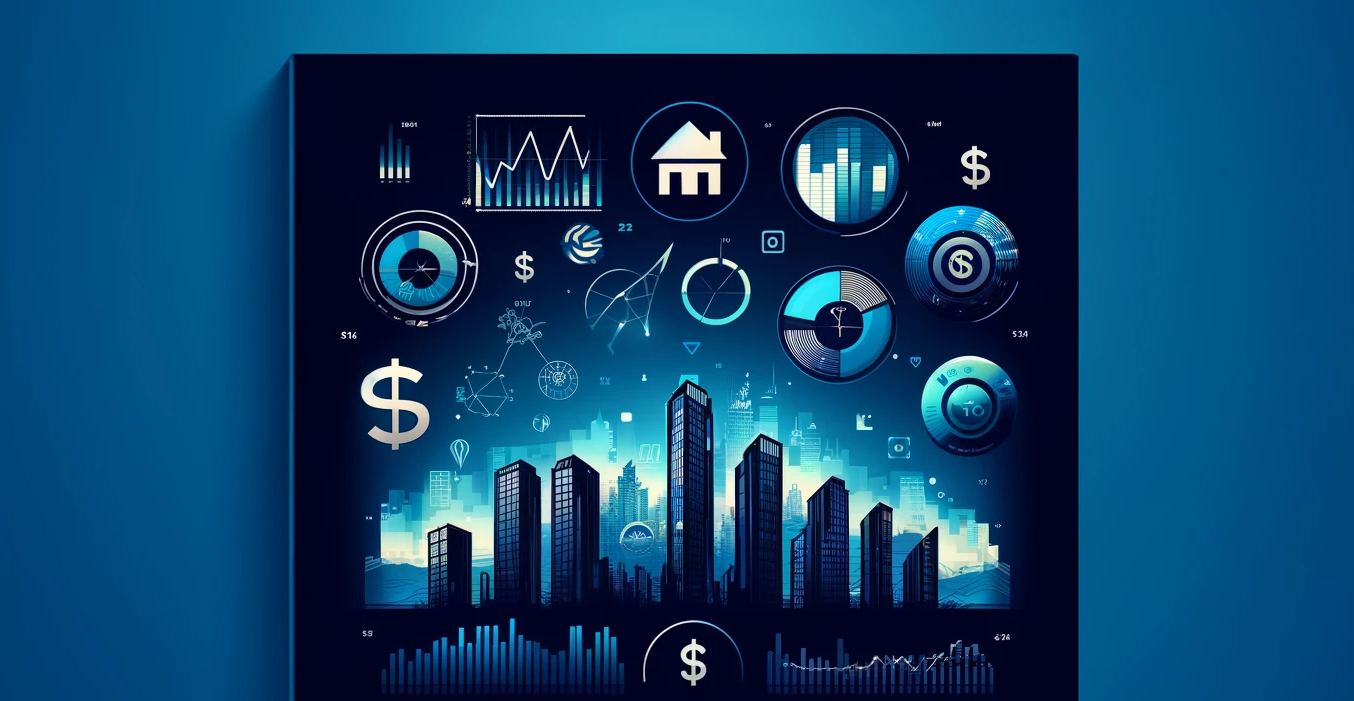
In this comprehensive study for Statistics E-109, I investigated the multifaceted influence of economic indicators such as housing starts, mortgage, and unemployment rates on the real estate price index. Through analysis utilizing datasets from the Federal Reserve Economic Data (FRED) and the S&P/Case-Shiller U.S. National Home Price Index, the project employs a multivariate regression model to decode the predictive power of these indicators. The findings offer insights for various stakeholders, from investors to policymakers, emphasizing the importance of economic conditions in real estate valuation.
This project serves as a testament to the intersection of real estate and economic indicators and stands as a blueprint for understanding the underlying dynamics shaping the housing market. The detailed study considers the implications of the results for real estate strategies and economic policymaking to enhance the resilience and stability of the housing market, as well as investment strategies.
Model Evaluation
The predictive power of the model was evaluated by comparing predicted real estate prices against actual prices. This step is critical for assessing the model's practical utility in forecasting market trends.
The "Predicted vs Actual" plot visualizes this comparison:
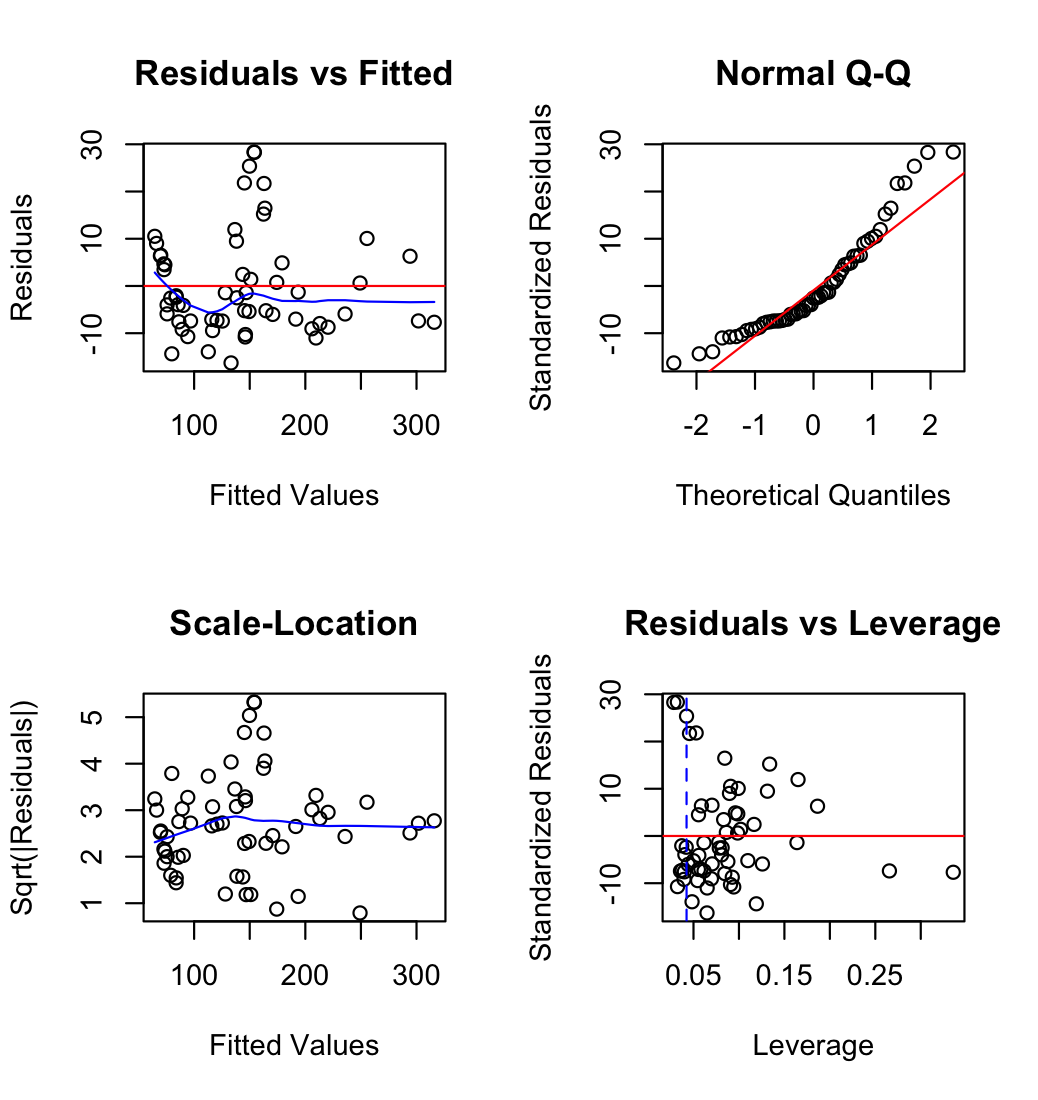
The congruence of the data points with the line of perfect prediction in the plot indicates the model's efficacy. While not perfect, as no model ever is, the consistency of the points along this line suggests that the model performs well in capturing the primary trends within the data.
To further refine our understanding, we look at measures such as the mean absolute error (MAE) and the root mean square error (RMSE), which provide additional context on the average deviation of the predictions from the actual values. Through these measures, we can quantify the model's accuracy in practical terms, furnishing both a validation of its strengths and a roadmap for improvement.
To further view the results of the study please review the README file in the GitHub repo linked below.
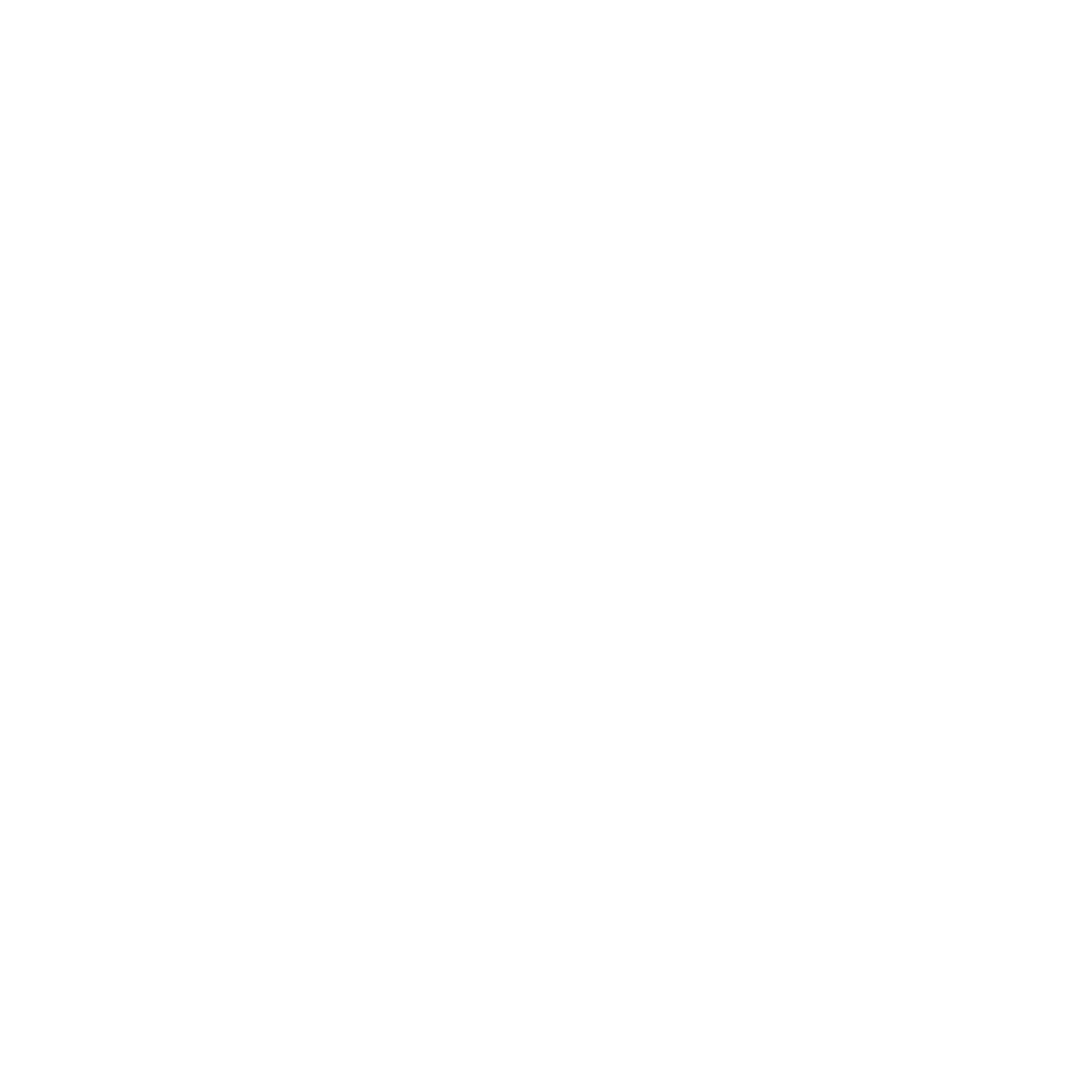